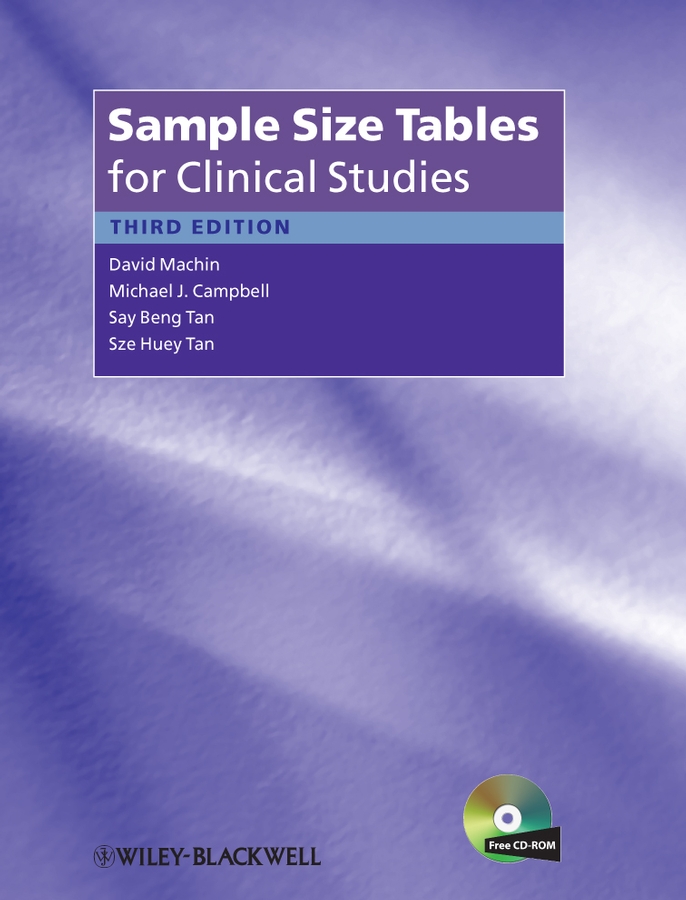
Contents
To
Oliver, Joshua and Sophie
David, John and Joseph
Lisa and Sophie
Kim San, Geok Yan and Janet
Companion CD-ROM
A companion CD-ROM is included with this book.
The CD contains software that will enable you to implement the sample size calculation
methods discussed in the book.
The CD content is referenced throughout the text where you see this symbol
See Chapter 17 for full details of the software on the CD and how to install and use it.
Technical requirements:
Operating System: Windows XP, 2000;
CPU: 500 MHz;
RAM: 128 MB;
Disc drive: 16 × CD drive;
Hard drive: 10 MB of free space
This edition first published 2009, © 2009 by D. Machin, M.J. Campbell, S.B. Tan, S.H. Tan
Previous editions: 1987, 1997
Blackwell Publishing was acquired by John Wiley & Sons in February 2007. Blackwell's publishing program has been merged with Wiley's global Scientific, Technical and Medical business to form Wiley-Blackwell.
Registered office: John Wiley & Sons Ltd, The Atrium, Southern Gate, Chichester, West Sussex, PO19 8SQ, UK
Editorial offices: 9600 Garsington Road, Oxford, OX4 2DQ, UK
The Atrium, Southern Gate, Chichester, West Sussex, PO19 8SQ, UK
111 River Street, Hoboken, NJ 07030-5774, USA
For details of our global editorial offices, for customer services and for information about how to apply for permission to reuse the copyright material in this book please see our website at
The right of the author to be identified as the author of this work has been asserted in accordance with the Copyright, Designs and Patents Act 1988.
All rights reserved. No part of this publication may be reproduced, stored in a retrieval system, or transmitted, in any form or by any means, electronic, mechanical, photocopying, recording or otherwise, except as permitted by the UK Copyright, Designs and Patents Act 1988, without the prior permission of the publisher.
Wiley also publishes its books in a variety of electronic formats. Some content that appears in print may not be available in electronic books.
Designations used by companies to distinguish their products are often claimed as trademarks. All brand names and product names used in this book are trade names, service marks, trademarks or registered trademarks of their respective owners. The publisher is not associated with any product or vendor mentioned in this book. This publication is designed to provide accurate and authoritative information in regard to the subject matter covered. It is sold on the understanding that the publisher is not engaged in rendering professional services. If professional advice or other expert assistance is required, the services of a competent professional should be sought.
The contents of this work are intended to further general scientific research, understanding, and discussion only and are not intended and should not be relied upon as recommending or promoting a specific method, diagnosis, or treatment by physicians for any particular patient. The publisher and the author make no representations or warranties with respect to the accuracy or completeness of the contents of this work and specifically disclaim all warranties, including without limitation any implied warranties of fitness for a particular purpose. In view of ongoing research, equipment modifications, changes in governmental regulations, and the constant flow of information relating to the use of medicines, equipment, and devices, the reader is urged to review and evaluate the information provided in the package insert or instructions for each medicine, equipment, or device for, among other things, any changes in the instructions or indication of usage and for added warnings and precautions. Readers should consult with a specialist where appropriate. The fact that an organization or Website is referred to in this work as a citation and/or a potential source of further information does not mean that the author or the publisher endorses the information the organization or Website may provide or recommendations it may make. Further, readers should be aware that Internet Websites listed in this work may have changed or disappeared between when this work was written and when it is read. No warranty may be created or extended by any promotional statements for this work. Neither the publisher nor the author shall be liable for any damages arising herefrom.
Library of Congress Cataloging-in-Publication Data
Sample size tables for clinical studies/David Machin . . . [et al.].—3rd ed. p.; cm.
Includes bibliographical references and index.
ISBN 978-1-4051-4650-0
1. Clinical trials—Statistical methods—Tables. I. Machin, David, 1939-
[DNLM: 1. Research Design—Tables. 2. Clinical Trials as Topic—Tables. 3. Sample Size—Tables.
4. Statistics as Topic—Tables. W 16 S192 2008]
R853. C55S36 2008
615.5072'4—dc22
2008030332
ISBN: 978-1-4051-4650-0
2008030332
Preface
It is now more than 20 years since the first edition of this book and 10 years from the second. The need for evidence-based estimates of the required size of a study is now universally recognized. Since the second edition the methodology for sample-size calculation has been widely extended, which is the main reason for a third edition. A second reason is the vastly improved computing power available. For the first edition, the tabulations were extensive to obviate separate calculations. A computer program to extend the range of the tables was available for the second edition.
This edition comes with sample size software , which we hope will give the user even greater flexibility and easy access to a wide range of designs, and allow design parameters to be tailored more readily to specific problems. Further, as some early phase designs are adaptive in nature and require knowledge of earlier patients’ response to determine the relevant options for the next patient, a (secure) database is provided for these.
Designing modern clinical research studies requires the involvement of multidisciplinary teams, with the process of sample size determination not being something that can be done by the statistician alone. So while software is available that can compute sample sizes (even from the internet), we feel that it is necessary that such software be complemented with a book that clearly explains and illustrates the methodology, along with tables. Feedback from users of earlier editions suggests that this can facilitate planning discussions within the research team.
Thus a major consideration has been to present the details, which are often complex, as clearly as possible and to illustrate these with appropriate examples. One objective of this approach is to encourage the wider use of sample size issues at the design stage in areas such as laboratory studies, which have been relatively neglected compared to epidemiological studies and clinical trials.
David Machin
Michael J. Campbell
Say Beng Tan
Sze Huey Tan
Singapore; Leicester and Sheffield, UK
1
Basic design considerations
SUMMARY
This chapter reviews the reasons why sample-size considerations are important when planning a clinical study of any type. The basic elements underlying this process including the null and alternative study hypotheses, effect size, statistical significance level and power are described. We introduce the notation to distinguish the population parameters we are trying to estimate from the study, from their anticipated value at the design stage, and finally their estimated value once the study has been completed. In the context of clinical trials, we emphasise the need for randomised allocation of subjects to treatment.
1.1 Why sample size calculations?
To motivate the statistical issues relevant to sample-size calculations, we will assume that we are planning a two-group clinical trial in which subjects are allocated at random to one of two alternative treatments for a particular medical condition and that a single binary endpoint (success or failure) has been specified in advance. However, it should be emphasised that the basic principles described, the formulae, sample-size tables and associated software included in this book are equally relevant to a wide range of design types covering all areas of medical research: ranging from the epidemiological, to clinical and laboratory-based studies.
Whatever the field of enquiry a well-designed study will have considered the questions posed carefully and, what is the particular focus for us, formally estimated the required sample size and will have recorded the supporting justification for the choice. Awareness of the importance of these has led to the major medical and related journals demanding that a detailed justification of the study size be included in any submitted article as it is a key component for peer reviewers to consider when assessing the scientific credibility of the work undertaken. For example, the General Statistical Checklist of the British Medical Journal, asks: ‘Was a pre-study calculation of study size reported?’
In any event, at a more mundane level, investigators, grant-awarding bodies and medical product development companies will all wish to know how much a study is likely to ‘cost’ both in terms of time and resource consumed as well as monetary terms. The projected study size will be a key component in this ‘cost’. They would also like to be reassured that the allocated resource will be well spent by assessing the likelihood that the study will give unequivocal results. In addition, the regulatory authorities, including the Food and Drug Administration (FDA 1988) in the USA and the Committee for Proprietary Medicinal Products (CPMP 1995) in the European Union, require information on planned study size. These are encapsulated in the guidelines of the International Conference on Harmonisation of Technical Requirements for Registration of Pharmaceuticals for Human Use (1998) ICH Topic E9.
If too few subjects are involved, the study is potentially a misuse of time because realistic medical differences are unlikely to be distinguished from chance variation. Too large a study can be a waste of important resources. Further, it may be argued that ethical considerations also enter into sample size calculations. Thus a small clinical trial with no chance of detecting a clinically useful difference between treatments is unfair to all the patients put to the (possible) risk and discomfort of the trial processes. A trial that is too large may be unfair if one treatment could have been ‘proven’ to be more effective with fewer patients as, a larger than necessary number of them has received the (now known) inferior treatment.
Providing a sample size for a study is not simply a matter of giving a single number from a set of tables. It is, and should be, a several-stage process. At the preliminary stages, what is required are ‘ball-park’ figures that enable the investigators to judge whether or not to start the detailed planning of the study. If a decision is made to proceed, then the later stages are to refine the supporting evidence for the early calculations until they make a persuasive case for the final patient numbers chosen which is then included (and justified) in the final study protocol.
Once the final sample size is determined, the protocol prepared and approved by the relevant bodies, it is incumbent on the research team to expedite the recruitment processes as much as possible, ensure the study is conducted to the highest of standards possible and eventually reported comprehensively.
Cautionary note
This book contains formulae for sample-size determination for many different situations. If these formulae are evaluated with the necessary input values provided they will give sample sizes to a mathematical accuracy of a single subject. However, the user should be aware that when planning a study of whatever type, one is planning in the presence of considerable uncertainty with respect to the eventual outcome. This suggests that, in the majority of applications, the number obtained should be rounded upwards to the nearest five, 10 or even more to establish the required sample size. We round upwards as that would give rise to narrower confidence intervals, and hence more ‘convincing’ evidence.
In some cases statistical research may improve the numerical accuracy of the formulae which depend on approximations (particularly in situations with small sample sizes resulting), but these improvements are likely to have less effect on the subsequent subject numbers obtained than changes in the planning values substituted into the formulae. As a consequence, we have specifically avoided using these refinements if they are computationally intensive. In contrast, and as appropriate, we do provide alternative methods which can easily be evaluated to give the design team a quick check on the accuracy of their computations and some reassurance on the output from and the tables we provide.
1.2 Design and analysis
Notation
In very brief terms the (statistical) objective of any study is to estimate from a sample the value of a population parameter. For example, if we were interested in the mean birth weight of babies born in a certain locality, then we may record the weight of a selected sample of n babies and their mean weight is taken as our estimate of the population mean birth weight denoted ωPop. The Greek ω distinguished the population value from its estimate Roman
. When planning a study, we are clearly ignorant of ωPop and neither do we have the data
. As we shall see later, when planning a study the investigators will usually need to provide some value for what ωPop may turn out to be. We term this anticipated value ωPlan. This value then forms (part of) the basis for subsequent sample size calculations. However, because adding ‘Plan’ as a subscript to the, often several, parameters concerned in the formulae for sample sizes included in this book, makes them even more cumbersome it is usually omitted, so ωPlan becomes simply ω. However to help with maintaining the distinction between ‘Plan’ and ‘Population’ values of parameters we have added the subscript ‘Pop’ to the latter. Unfortunately, although making subsequent chapters easier, this rather complicates the sections immediately below.
The randomised controlled trial
Consider, as an example, a proposed randomised trial of a placebo (control) against acupuncture in the relief of pain in a particular diagnosis. The patients are randomised to receive either placebo or acupuncture (how placebo acupuncture can be administered is clearly an important consideration). In addition, we assume that pain relief is assessed at a fixed time after randomisation and is defined in such a way as to be unambiguously evaluable for each patient as either ‘success’ or ‘failure’. We assume the aim of the trial is to estimate the true difference δPop between the true success rate πPopA of Acupuncture and the true success rate πPopC of Control. Thus the key (population) parameter of interest is δPop which is a composite of the two (population) parameters πPopA and πPopC.
At the completion of the trial the Acupuncture group of patients yield a treatment success rate pA which is an estimate of πPopA and the Control group give success rate pC which is an estimate of πPopC. Thus, the observed difference, d = pA – pC, provides an estimate of the true difference δPop = πPopA – πPopC.
In contrast, at the design stage of the trial one can only postulate what the size of difference (strictly the minimum size of interest) might be and we denote this by δPlan.
The number of patients necessary to recruit to a particular study depends on:
The null and alternative hypotheses, and effect size
Null hypothesis
In our example, the null hypothesis, termed HNull, implies that acupuncture and placebo are equally effective or that πPopA = πPopC. Even when that null hypothesis is true, observed differences, d = pA – pC other than zero, will occur. The probability of obtaining the observed difference d or a more extreme one given that πPopA = πPopC can be calculated. If, under this null hypothesis, the resulting probability or p-value was very small, then we would reject the null hypothesis. We then conclude the two treatments do indeed differ in efficacy.
Alternative hypothesis
Usually in statistical significance testing, by rejecting the null hypothesis, we do not specifically accept any alternative hypothesis and it is usual to report the range of plausible population values with a confidence interval (CI). However, sample-size calculations are usually posed in a hypothesis test framework, and this requires us to specify an alternative hypothesis, termed HAlt, that is, πPopA – πPopC = δPop with δPop ≠ 0. The value δPop is known as the true effect size.
Establishing the effect size
Of the parameters that have to be pre-specified before the sample size can be determined, the true effect size is the most critical and, in order to estimate sample size, one must first identify the magnitude of the difference one wishes to detect by means of δPlan.
Sometimes there is prior knowledge that enables an investigator to anticipate what treatment benefit is likely to be observed, and the role of the trial is to confirm that expectation. At other times it may be possible to say that, for example, only the prospect of doubling of their median survival would be worthwhile for patients with this type of rapidly fatal disease because the new treatment is so toxic.
One additional problem is that investigators are often optimistic about the effect of new treatments; it can take considerable effort to initiate a trial and so, in many cases, the trial would only be launched if the investigator is enthusiastic about the new treatment and is sufficiently convinced about its potential efficacy. Experience suggests that as trials progress there is often a growing realism that, even at best, the initial expectations were optimistic and there is ample historical evidence to suggest that trials which set out to detect large treatment differences nearly always result in ‘no significant difference was detected’. In such cases there may have been a true and worthwhile treatment benefit that has been missed, since the level of detectable differences set by the design was unrealistically high, and hence the sample size too small.
In practice a form of iteration is often used. The clinician team might offer a variety of opinions as to what clinically useful difference will transpire—ranging perhaps from the unduly pessimistic small effect to the optimistic (and unlikely in many situations) large effect. Sample sizes may then be calculated under this range of scenarios with corresponding patient numbers ranging perhaps from extremely large to the relatively small. The importance of the clinical question, and/or the impossibility of recruiting large patient numbers may rule out a very large trial but to conduct a small trial may leave important clinical effects not firmly established. As a consequence, the team may next define a revised aim maybe using a summary derived from the original opinions, and the calculations are repeated. Perhaps the sample size now becomes attainable and forms the basis for the definitive protocol.
There are a number of ways of eliciting useful effect sizes: a Bayesian perspective has been advocated by Spiegelhalter, Freedman and Parmar (1994), an economic approach by Drummond and O’Brien (1993) and one based on patients’ perceptions rather than clinicians’ perceptions of benefit by Naylor and Llewellyn-Thomas (1994).
Test size, significance level or Type I error
The critical value we take for the p-value is arbitrary, and we denote it by α. If p-value ≤ α one rejects the null hypothesis, conversely if p-value > α one does not reject the null hypothesis. Even when the null hypothesis is in fact true there is a risk of rejecting it and to reject the null hypothesis when it is true is to make a Type I error. The probability of rejecting the null hypothesis when it is true is α. The quantity α can be referred to either as the test size, significance level, probability of a Type I error or the false-positive error. Conventionally α = 0.05 is often used.
Type II error and power
The clinical trial could yield an observed difference d that would lead to a p-value > α even though the null hypothesis is really not true, that is, πPopA truly differs from πPopC. In such a situation, we then fail to reject the null hypothesis when it is in fact false. This is called a Type II or false-negative error and the probability of this is denoted by β.
The probability of a Type II error is based on the assumption that the null hypothesis is not true, that is, δPop = πPopA – πPopC ≠ 0. There are clearly many possible values of δPop in this instance since many values other than zero satisfy this condition, and each would give a different value for β.
The power is defined as one minus the probability of a Type II error, 1 – β. That is, ‘power’ is the probability of obtaining a ‘significant’ p-value if the null hypothesis is really false. Conventionally a minimum power of 80% is required in a clinical trial.
One and two-sided significance tests
It is usual for most clinical trials that there is considerable uncertainty about the relative merits of the alternative treatments so that even when the new treatment or intervention under test is thought for scientific reasons to be an improvement over the current standard, the possibility that this is not the case is allowed for. For example, in the clinical trial conducted by Chow, Tai, Tan et al. (2002) it was thought at the planning stage that high dose tamoxifen would improve survival over placebo in patients with inoperable hepatocellular carcinoma. This turned out not to be the case and, if anything, tamoxifen was detrimental to the ultimate survival. This is not an isolated example.
Since it is plausible to assume in the acupuncture trial referred to earlier that the placebo is in some sense ‘inactive’ and that any ‘active’ treatment will have to perform better than the ‘inactive’ treatment if it is to be adopted into clinical practice, then the alternative hypothesis may be that the acupuncture has an improved success rate, that is, πPopA > πPopC. This leads to a one-sided or one-tailed statistical significance test.
On the other hand, if we cannot make this type of assumption about the new treatment at the design stage, then the alternative hypothesis is that πPopA and πPopC differ, that is, πPopA ≠ πPopC.
In general, for a given sample size, a one-sided test is more powerful than the corresponding two-sided test. However, a decision to use a one-sided test should never be made after looking at the data and observing the direction of the departure. Such decisions should be made at the design stage and one should use a one-sided test only if it is certain that departures in the particular direction not anticipated will always be ascribed to chance, and therefore regarded as non-significant, however large they are. It will almost always be preferable to carry out two-sided hypothesis tests but, if a one-sided test is to be used, this should be indicated and justified for the problem in hand.
Confidence intervals
Medical statisticians often point out that there is an over-emphasis on tests of significance in the reporting of results and they argue that, wherever possible, confidence intervals (CI) should be quoted (see Chapter 2). The reason for this is that a p-value alone gives the reader, who wishes to make use of the published results of a particular trial, little practical information. In contrast, quoting an estimate of the effect with the corresponding (usually 95%) confidence interval, enables him or her to better judge the relative efficacy of the alternative treatments. For the purposes of this book, the associated software and in the planning stages of the trial, discussion is easier in terms of statistical significance but nevertheless it should be emphasised that key confidence intervals should always be quoted in the final report of any study of whatever design.
Randomisation
As Machin and Campbell (2005) and many others point out, of fundamental importance to the design of any clinical trial (and to all types of other studies when feasible) is the random allocation of subjects to the options under study. Such allocation safeguards in particular against bias in the estimate of group differences and is the necessary basis for the subsequent statistical tests.
1.3 Practicalities
Power and significance tests
In a clinical trial, two or more forms of therapy or intervention may be compared. However, patients themselves vary both in their baseline characteristics at diagnosis and in their response to subsequent therapy. Hence in a clinical trial, an apparent difference in treatments may be observed due to chance alone, that is, we may observe a difference but it may be explained by the intrinsic characteristics of the patients themselves rather than ‘caused’ by the different treatments given. As a consequence, it is customary to use a ‘significance test’ to assess the weight of evidence and to estimate the probability that the observed data could in fact have arisen purely by chance. The results of the significance test, calculated on the assumption that the null hypothesis is true, will be expressed as a ‘p-value’. For example, at the end of the trial if the difference between treatments is tested, then a p < 0.05 would indicate that so extreme an observed difference could be expected to have arisen by chance alone less than 5% of the time, and so it is quite likely that a treatment difference really is present.
However, if only a few patients were entered into the trial then, even if there really were a true treatment difference, the results are less convincing than if a much larger number of patients had been assessed. Thus, the weight of evidence in favour of concluding that there is a treatment effect will be much less in a small trial than in a large one. In statistical terms, we would say that the ‘sample size’ is too small, and that the ‘power of the test’ is very low.
The ‘power’ of a significance test is a measure of how likely a test is to produce a statistically significant result, given a true difference between the treatments of a certain magnitude.
Sample size and interpretation of significance
Suppose the results of an observed treatment difference in a clinical trial are declared ‘not statistically significant’. Such a statement only indicates that there was insufficient weight of evidence to be able to declare: ‘that the observed difference is unlikely to have arisen by chance’. It does not imply that there is ‘no clinically important difference between the treatments’ as, for example, if the sample size was too small the trial might be very unlikely to obtain a significant p-value even when a clinically relevant difference is truly present. Hence it is of crucial importance to consider sample size and power when interpreting statements about ‘non-significant’ results. In particular, if the power of the test was very low, all one can conclude from a non-significant result is that the question of treatment differences remains unresolved.
Estimation of sample size and power
In estimating the number of patients required for a trial (sample size), it is usual to identify a single major outcome which is regarded as the primary endpoint for comparing treatment differences. In many clinical trials this will be a measure such as response rate, time to wound healing, degree of palliation, or a quality of life index.
It is customary to start by specifying the size of the difference required to be detected, and then to estimate the number of patients necessary to enable the trial to detect this difference if it truly exists. Thus, for example, it might be anticipated that acupuncture could improve the response rate from 20 to 30%, and that since this is a plausible and medically important improvement, it is desired to be reasonably certain of detecting such a difference if it really exists. ‘Detecting a difference’ is usually taken to mean ‘obtain a statistically significant difference with p-value < 0.05’; and similarly the phrase ‘to be reasonably certain’ is usually interpreted to mean something like ‘have a chance of at least 90% of obtaining such a p-value’ if there really is an improvement from 20 to 30%. This latter statement corresponds, in statistical terms, to saying that the power of the trial should be 0.9 or 90%.
More than one primary outcome
We have based the above discussion on the assumption that there is a single identifiable end point or outcome, upon which treatment comparisons are based. However, often there is more than one endpoint of interest within the same trial, such as wound healing time, pain levels and methicillin-resistant Staphylococcus aureus (MRSA) infection rates. If one of these endpoints is regarded as more important than the others, it can be named as the primary endpoint and sample-size estimates calculated accordingly. A problem arises when there are several outcome measures which are all regarded as equally important. A commonly adopted approach is to repeat the sample-size estimates for each outcome measure in turn, and then select the largest number as the sample size required to answer all the questions of interest.
Here, it is essential to note the relationship between significance tests and power as it is well recognised that p-values become distorted if many endpoints (from the same patients) are each tested for significance. Often a smaller p-value will be considered necessary for statistical significance to compensate for this. In such cases, the sample-size calculations will use the reduced test size and hence increase the corresponding study size.
Internal pilot studies
In order to calculate the sample size for a trial one must first have available some background information. For example, for a trial using a survival endpoint one must provide the anticipated survival of the control group. Also, one must have some idea as to what is a realistic difference to seek. Sometimes such information is available as rather firm prior knowledge from the work of others, at other times, a pilot study may be conducted to obtain the relevant information.
Traditionally, a pilot study is a distinct preliminary investigation, conducted before embarking on the main trial but several authors, including Browne (1995), have advocated the use of an internal pilot study. The idea here is to plan the clinical trial on the basis of the best (current) available information, but to regard the first patients entered as the internal pilot. When data from these patients have been collected, the sample size can be re-estimated with the revised knowledge that the data from these first patients have provided. Two vital features accompany this approach: firstly, the final sample size should only ever be adjusted upwards, never down; and secondly, one should only use the internal pilot information in order to improve the design features which are independent of the treatment variable. This second point is crucial. It means that, for example, if treatments are to be compared using a t-test, then a basic ingredient of the sample-size calculation will be the standard deviation (σPlan) whose value may be amended following the pilot phase and then potentially used to revise upwards the ultimate sample size. No note of the observed difference (the effect) between treatments is made so that δPlan remains unchanged in the revised calculations.
The advantage of an internal pilot is that it can be relatively large—perhaps half of the anticipated patients. It provides an insurance against misjudgement regarding the baseline planning assumptions. It is, nevertheless, important that the intention to conduct an internal pilot study is recorded at the outset and that full details are given in the study protocol.
More than two groups
The majority of clinical trials involve a simple comparison between two interventions or treatments. When there are more than two treatments the situation is much more complicated. This is because there is no longer one clear alternative hypothesis. Thus, for example, with three groups, although the null hypothesis is that the population means are all equal, there are several potential alternative hypotheses. These include one which postulates that two of the group means are equal but which differ from the third, or one that the means are ordered in some way. Alternatively the investigators may simply wish to compare all three groups, leading to three pairwise comparisons which may not all be equally important.
One problem arising at the time of analysis is that such situations may lead to multiple significance tests, resulting in misleading p-values. Various solutions have been proposed, each resulting in different analysis strategies and therefore different design and sample size considerations. One approach that is commonly advocated is to conduct an analysis of variance (ANOVA) or a similar global statistical test, with pairwise or other comparisons of means only being made if the global test is significant. Another approach is to use conventional significance tests but with an adjusted significance level obtained from the Bonferroni correction––essentially reducing the conventional test size (say, 0.05) by dividing by the number of comparisons to be made. However, the simplest strategy is to adopt the approach which regards, for example, a three-treatment groups comparison as little different from carrying out a series of three independent trials, and to use conventional significance tests without adjustment as argued by Saville (1990). As a consequence, and assuming equal numbers of subjects per treatment arm, the sample size is first estimated for the three distinct trial comparisons. Then for each treatment group simply take the maximum of these as the sample size required.
Studies with g (> 2) groups may compare different doses of the same therapy or some other type of ordered treatment groups. Thus, although the null hypothesis would still be that all population means are equal, the alternative will now be HOrdered which is either μPop1 < μPop1 < . . . < μPop1 or μPop1 > μPop1 > . . . > μPopg. In the simplest case, the doses may be equally spaced either on the original or possibly a logarithmic scale, and these may allow HOrdered to be expressed as μPop = αPop + βPop (dose). The study is then designed to estimate the regression coefficient, βPop, and the sample size is calculated on the basis of an anticipated value, βPlan
A rather different situation arises with factorial designs. Suppose that a 2 × 2 factorial trial is planned to compare two factors, A and B each of two levels, then there will be four groups to be compared with m subjects per group. The design may be particularly useful in circumstances where (say) factor A addresses a major therapeutic question, while factor B poses a more secondary one. For example, A might be the addition of a further drug to an established combination chemotherapy for a cancer while B may the choice of anti-emetic delivered with the drugs. For efficient use of such a design the two main effects, that is the different options within A and those within B, are compared using two means with 2m subjects in each group. However, this assumes an absence of interaction between the factors which means that the effect of A remains the same irrespective of which of the options within B the patient receives and vice-versa. If this is not the case, we might then wish to estimate the size of this interaction effect and so have a sufficiently large sample size for this purpose.
In planning a 2 × 2 factorial trial, the first step would be to assume no interaction was present and consider the sample size for factor A. The second step would be to consider the sample size for factor B which may have a different effect size, test size and power, from the factor A comparison. Clearly, if the resulting sample sizes are similar then there is no difficulty in choosing, perhaps the larger, as the required sample size. If the sample sizes are very disparate then a discussion would ensue as to the most important comparison and perhaps a reasonable compromise reached. This compromise figure could then be used to check what magnitude of interaction (if present) could be detected with such numbers and may have to be increased if there is a strong possibility of an interaction being present.
Rules of thumb
Although we provide in later chapters methods of determining sample sizes in a variety of contexts, it is often very useful (especially at initial planning meetings) to have a ‘feel’ of the order of magnitude of the sample size that may ultimately be required. Thus some ‘rules of thumb’ are given in the appropriate chapters for this purpose while Van Belle (2002) provides a more comprehensive review.
1.4 Use of tables and software
It is hoped that the tables and the associated software will prove useful in a number of ways.
Number of subjects
Before conducting a clinical trial to test the value of acupuncture a researcher believes that the placebo group will yield a response rate of 30%. How many subjects are required to demonstrate an anticipated response rate for acupuncture of 70% at a given significance level and power?
Power of a study
A common situation is one where the number of patients is governed by forces such as time, money, human resources and disease incidence rather than by purely scientific criteria. The researcher may then wish to know what probability he or she has of detecting a certain difference in treatment efficacy with a trial of the intended size.
Size of effect
In this case, the sample size is constrained, and the researcher is interested in exploring the size of effects which could be established for a reasonable power, say, 80%.
1.5 The protocol
As we have indicated the justification of sample size in any study is important. This not only gives an indication of the resources required but also forces the research team to think about issues of design carefully. We give below examples of how the resulting calculations were justified.
Example 1.1—surgical resection for patients with gastric cancer
Cuschieri, Weeden, Fielding et al. (1999) compared two forms of surgical resection for patients with gastric cancer. The primary outcome (event of interest) was time to death. The authors state:
‘Sample size calculations were based on a pre-study survey of 26 gastric surgeons, which indicated that the baseline 5-year survival rate of D1 surgery was expected to be 20%, and an improvement in survival to 34% (14% change) with D2 resection would be a realistic expectation. Thus 400 patients (200 in each arm) were to be randomised, providing 90% power to detect such a difference with p-value < 0.05’.
Example 1.2—steroid or cyclopsporine for oral lichen planus
The protocol of March 1998 of the subsequently published trial conducted by Poon, Goh, Kim et al. (2006) to compare steroid with cyclosporine for the topical treatment of oral lichen planus stated:
‘It is anticipated that in patients taking topical steroids, the response rate at 1 month will be approximately 60%. It is anticipated that this may be raised to as much as 80% in those receiving cyclosporine. With two-sided test size 5%, power 80%, then the corresponding number of patients required is approximately 200 (Machin, Campbell, Fayers and Pinol 1997, ).’
Example 1.3—sequential hormonal therapy in advanced and metastatic breast cancer
Iaffaioli, Formato, Tortoriello et al. (2005) conducted two Phase II trials of sequential hormonal therapy with first-line anastrozole and with second-line exemestane, in advanced and metastatic breast cancer. This example is discussed further in Chapter 17.
The authors provide their justification for sample size as follows (we just show the justification for the anastrozole study, a similar justification was provided for the exemestane study):
‘The sample size calculation for both single-stage studies was performed as proposed by A’Hern (2001), this method being an exact version of the algorithm first presented by Fleming (1982). The anastrizole evaluation required 93 subjects to decide whether the proportion of patients with a clinical benefit (P) was ≤ 50% or ≥ 65%. If the number of patients with clinical benefit was ≥ 55, the hypothesis that P ≤ 50% was rejected with a target error rate of 0.050 and an actual error rate of 0.048. If the number of patients with clinical benefit was ≤ 54, the hypothesis that P ≥ 65% was rejected with a target error rate of 0.100 and an actual error rate of 0.099.’
1.6 Books on sample-size calculations
Chow SC, Shao J and Wang H (2008). Sample Size Calculations in Clinical Research, 2nd edn. Marcel Dekker, New York.
Cohen J (1988). Statistical Power Analysis for the Behavioral Sciences, 2nd edn. Lawrence Earlbaum, New Jersey.
Lemeshow S, Hosmar DW, Klar J and Lwanga SK (1990). Adequacy of Sample Size in Health Studies. John Wiley & Sons, Chichester.
Lipsey MW (1990). Design Sensitivity: Statistical Power for Experimental Research. Sage Publications, London.
Machin D and Campbell MJ (2005). Design of Studies for Medical Research, John Wiley & Sons, Chichester.
Schuster JJ (1993). Practical Handbook of Sample Size Guidelines for Clinical Trials. CRC Press, FL.
1.7 Software for sample-size calculations
Since sample-size determination is such a critical part of the design process we recommend that all calculations are carefully checked before the final decisions are made. This is particularly important for large and/or resource intensive studies. In-house checking by colleagues is also important.
Biostat (2001). Power & Precision: Release 2.1. Englewood, NJ.
Lenth RV (2006). Java Applets for Power and Sample Size. URL: .
National Council for Social Studies (2005). Power Analysis and Sample Size Software (PASS): Version 2005. NCSS Statistical Software, Kaysville, UT.
SAS Institute (2004). Getting Started with the SAS Power and Sample Size Application: Version 9.1. SAS Institute, Cary, NC.
StataCorp (2007). Stata Statistical Software: Release 10. College Station, TX.
Statistical Solutions (2006). nQuery Adviser: Version 6.0. Saugus, MA.
1.8 References
A’Hern RP (2001). Sample size tables for exact single stage phase II designs. Statistics in Medicine, 20, 859–866.
Browne RH (1995). On the use of a pilot study for sample size determination. Statistics in Medicine, 14, 1933–1940.
Chow PK-H, Tai B-C, Tan C-K, Machin D, Johnson PJ, Khin M-W and Soo K-C (2002). No role for high-dose tamoxifen in the treatment of inoperable hepatocellular carcinoma: An Asia-Pacific double-blind randomised controlled trial. Hepatology, 36, 1221–1226.
CPMP Working Party on Efficacy of Medicinal Products (1995). Biostatistical methodology in clinical trials in applications for marketing authorizations for medical products. Statistics in Medicine, 14, 1659–1682.
Cuschieri A, Weeden S, Fielding J, Bancewicz J, Craven J, Joypaul V, Sydes M and Fayers P (1999). Patient survival after D1 and D2 resections for gastric cancer: long-term results of the MRC randomized surgical trial. British Journal of Cancer, 79, 1522–1530.
Drummond M and O’Brien B (1993). Clinical importance, statistical significance and the assessment of economic and quality-of-life outcomes. Health Economics, 2, 205–212.
FDA (1988). Guidelines for the Format and Content of the Clinical and Statistics Section of New Drug Applications. US Department of Health and Human Services, Public Health Service, Food and Drug Administration, Washington D.C.
Fleming TR (1982). One-sample multiple testing procedure for Phase II clinical trial. Biometrics, 38, 143–151.
Iaffaioli RV, Formato R, Tortoriello A, Del Prete S, Caraglia M, Pappagallo G, Pisano A, Fanelli F, Ianniello G, Cigolari S, Pizza C, Marano O, Pezzella G, Pedicini T, Febbraro A, Incoronato P, Manzione L, Ferrari E, Marzano N, Quattrin S, Pisconti S, Nasti G, Giotta G, Colucci G and other Goim authors (2005) Phase II study of sequential hormonal therapy with anastrozole/exemestane in advanced and metastatic breast cancer. British Journal of Cancer, 92, 1621–1625.
International Conference on Harmonisation of Technical Requirements for Registration of Pharmaceuticals for Human Use (1998). Statistical Principles for Clinical Trials E9. Available at .
Machin D and Campbell MJ (2005). Design of Studies for Medical Research. John Wiley & Sons, Chichester.
Machin D, Campbell MJ, Fayers PM and Pinol A (1997). Statistical Tables for the Design of Clinical Studies, 2nd edn. Blackwell Scientific Publications, Oxford.
Naylor CD and Llewellyn-Thomas HA (1994). Can there be a more patients-centred approach to determining clinically important effect size for randomized treatments? Journal of Clinical Epidemiology, 47, 787–795.
Poon CY, Goh BT, Kim M-J, Rajaseharan A, Ahmed S, Thongsprasom K, Chaimusik M, Suresh S, Machin D, Wong-HB and Seldrup J (2006). A randomised controlled trial to compare steroid with cyclosporine for the topical treatment of oral lichen planus. Oral Surgery, Oral Medicine, Oral Pathology, Oral Radiolology and Endodontics, 102, 47–55.
Saville DJ (1990). Multiple comparison procedures: The practical solution. The American Statistician, 44, 174–180.
Speigelhalter DJ, Freedman LS and Parmar MKB (1994). Bayesian approaches to randomized trials (with discussion). Journal of the Royal Statistical Society (A), 157, 357–416.
Van Belle G (2002). Statistical Rules of Thumb. John Wiley & Sons, Chichester.